When knitting met machine learning, an untold love story.
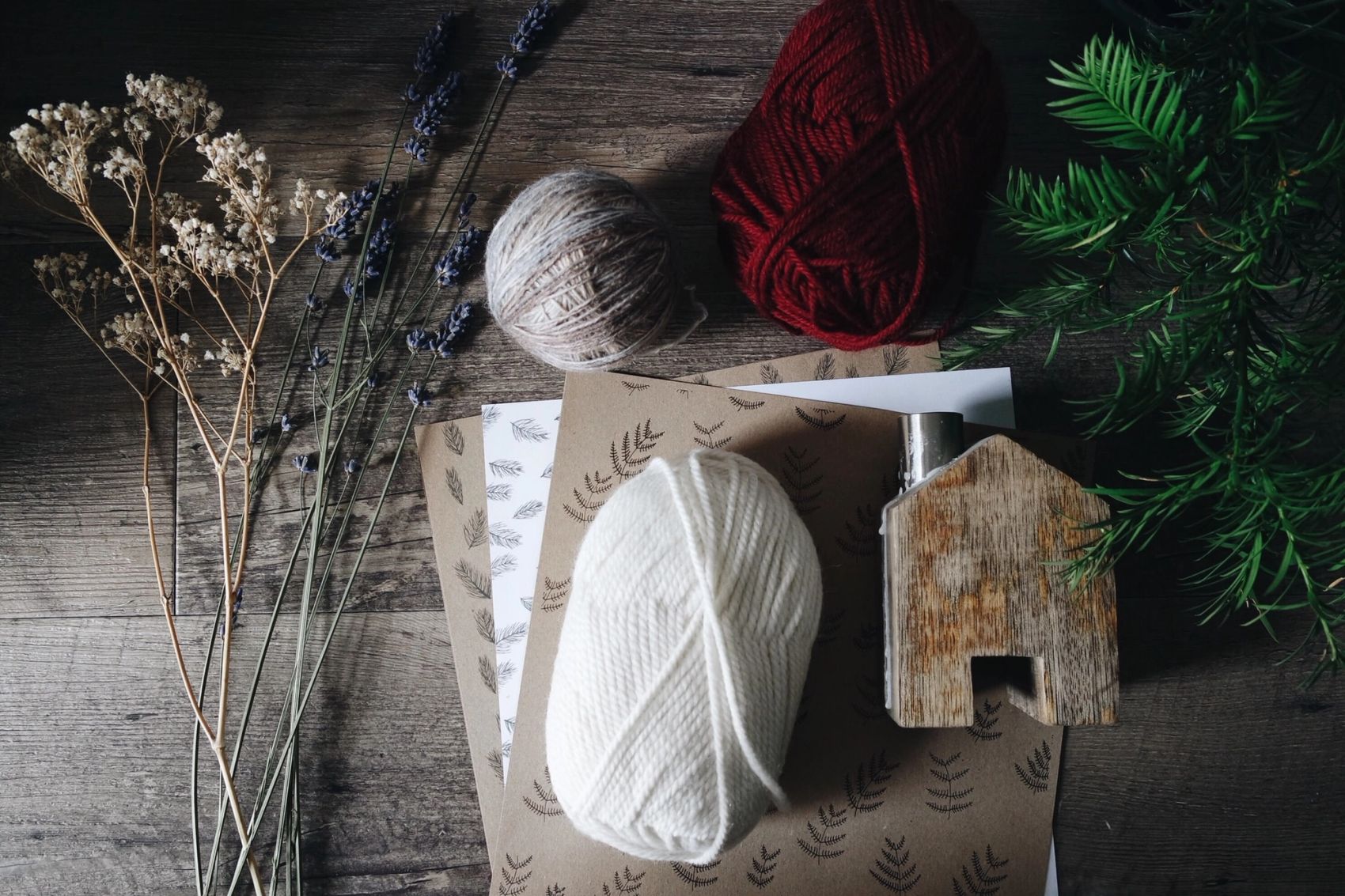
You heard it here first: machine learning and knitting are officially in a relationship! Yes, knitting, the act of transforming yarn into textile and fabric.
Thanks to a group of researchers at the Massachusetts Institute of Technology, there now exists software which when fed a picture of a garnet, produces instructions for that exact pattern, used by automated knitting machines – they call it Inverse knitting. While on the subject, they are also the brains behind the project Knitting skeletons, a software which lets you design your own knitwear, without any knitting or coding experience required. Amazing!
Let's take a look at Inverse knitting!
Today's standard is using advanced knitting machines for mass production of garnets and textiles. However, these require pre designed recipes, and are maintained by low-level programming code, hence requiring a certain expertise to develop and maintain. This was the motivation behind the development of Neural Inverse Knitting; the intersection between machine learning and knitting.
So how does one combine machine learning and knitting? You might consider this an unlikely combination, however, there is a logical approach behind the problem. In essence, this is a problem within pattern recognition - a common problem in the world of machine learning.
The solution presented in the article employs methods such as generative adversarial networks (GAN), and convolutional neural networks (CNN) to automate the generation of knitting patterns for industrial use. In particular, GAN was applied to generate artificial textile samples used for training the network, whereas CNN is used to generate the main image-to-recipe-model, named Img2Prog. We will proceed by looking at the steps to solving this unconventional combination of fields.

- First, a knitting language is defined, describing knitting patterns. This knitting language consists of 17 operations, and is possibly recognisable by knitting enthusiasts. Here we find operations such as knit, purl, tuck, and miss to name a few. The rest of the operations are listed in the figure above.
- Second, we need training data. For this particular case, the training data consisted of several pictures of patterned clothing. However, after collecting over 2,000 real photographs of patterns from clothing and textiles, the authors realised they had to resort to artificial data. In combination with real data, artificial data plays a strong role in data science and machine learning, and is both quick and inexpensive to produce. To overcome the current challenge, the real data was melded with the synthetic images, to create regularised synthetic images of knitting patterns, using GAN. This way there was a consistency between the real and artificial data.
- Finally, the model, a prediction network, was trained using CNN. For reference, the entire process is visualised in the figure below. The resulting predictor, named Img2Prog, could now be fed an image of a pattern as input, producing the recipe for the corresponding pattern as output. Amazing!

If you still have some Christmas gifts left to buy/make, you now know what to do. Find your favourite theme, take a picture, apply Img2Prog and start knitting! Merry Knitmas :D